Marvin Minsky Didn’t Live To See Artificial General Intelligence. You Might Not Either. - Unpublished, January 2016
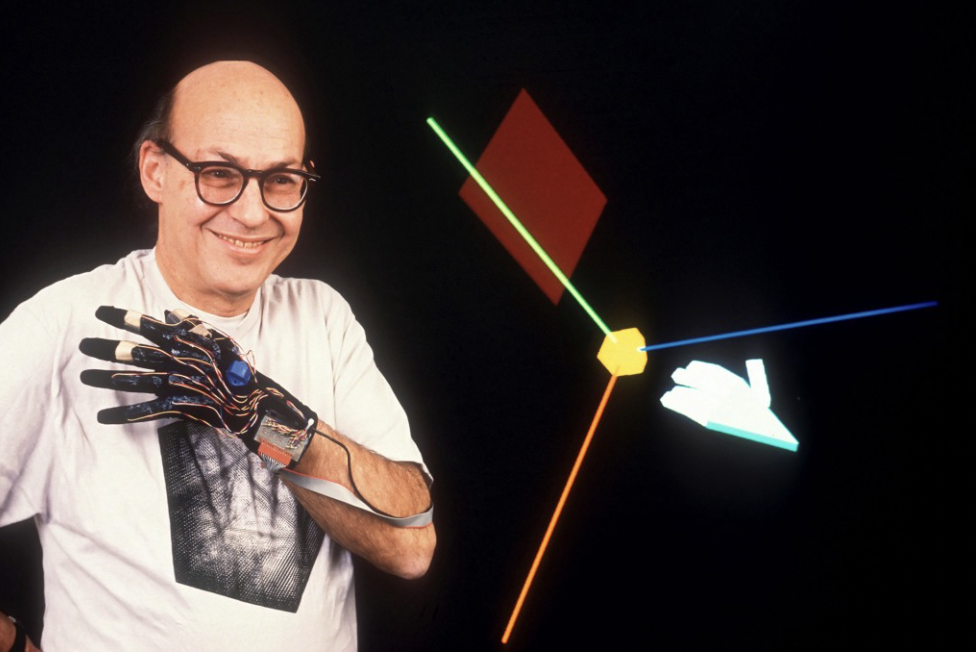
As we mourn the loss of Marvin Minsky, one of the founding fathers of Artificial Intelligence, let us look at the significance of what he started. AI is an undertaking that should be considered one of the most significant attempts to improve the human condition. Yet, much more of today’s collective intelligence is spent designing advertising on social media than on the advancement of humanity through AI. Why have we been so ineffective at solving the challenges set fourth by Minsky and his colleagues 60 years ago? Let us look at technical challenges and the broken incentive structures that limit our success and find out how AI research could potentially dream bigger than improving the data processing applications of today.
___
Marvin Minsky was perhaps the most influential of the researchers attending the now famous Summer Research Project on Artificial Intelligence (1956). Organized by Dartmouth’s John McCarthy, the summer program brought together leading minds to develop ‘artificial intelligence’, during which this term was first coined.
“We propose that a 2 month, 10 man study of ‘artificial intelligence’ be carried out during the summer of 1956 at Dartmouth College. The study is to proceed on the basis of the conjecture that every aspect of learning or any other feature of intelligence can in principle be so precisely described that a machine can be made to simulate it. An attempt will be made to find how to make machines use language, form abstractions and concepts, solve kinds of problems now reserved for humans, and improve themselves. We think that a significant advance can be made in one or more of these problems if a carefully selected group of scientists work on it together for a summer.”
Extract from Founding Statement of Dartmouth Conference, McCarthy et al, 1955
Minsky’s and McCarthy’s belief that “every aspect of learning or any other feature of intelligence” could be simulated by any form of an agent other than a human one is a striking statement in its implication: What would happen if this was achieved? The statement is also striking in another capacity: The founders of Artificial Intelligence were ambitious (or, to some, naive) enough to think that a “significant advance” could be made by ten researchers in the course of a summer. 60 years later, researchers are still striving towards making such a significant advance. And — So what? you might query. Why should we care?
Minsky’s Dream
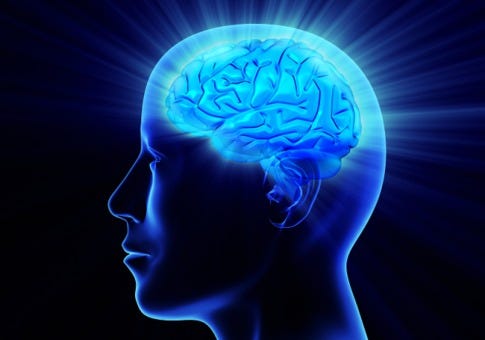
Artificial Intelligence may be a problem like no other, but it certainly holds a promise like nothing else. For all problems faced by modern humans, for the challenges that we have to meet as a global civilization and for all questions in the sciences, intelligence is the most important tool we can apply. If we could broaden the range and depth of the spectrum of intelligence, we could dramatically extend the range of problems we can successfully address. We can think of AI as a Meta-Science, one that has the capabilities to further every other scientific and practical endeavor. It is a horizontal, not a vertical, innovation.
“What we want to do is work toward things like cures for human diseases, immortality, the end of war. These problems are too huge for us to tackle today. The only way is to get smarter as a species—through evolution or genetic engineering, or through A.I.”
Doug Lenat, CYC Founder
Marvin Minsky, like most of the founding fathers of AI, had a dream. The dream was to build human-level (and above) artificial intelligence. What happens if we could simulate intelligence in machines to problem-solve for us? The range of intelligent capabilities would certainly be broadened, because we can expect machine intelligence to differ from our own brains. AI solvers for games such as Chess and GO are able to show moves we might not have previously considered. Imagine tools like these doing hypothesis generation for scientific research. Imagine, in this way, machine intelligence applied to fundamental physics. And, of course, machine intelligence working on the improvement of machine intelligence itself — imagine the increase in the power of AI that could rise out of such multipliers!
The creation of some form of ultra machine intelligence was the dream of many AI researchers including Minsky. Such an intelligence would autonomously assess the data points currently defining a problem, and conquer the space of its possible solutions in ways no human individual or collective could. As I.J. Good stated in 1965, “The first ultraintelligent machine is the last invention that man need ever make.” These ideas still seem far fetched, certainly above and beyond what McCarthy et al. set out to achieve at the Dartmouth Conference in 1956.
In reality, these ideas are fathomable. Despite our lack of success in recreating human intelligence, a wide range of problem-solvers utilizing machine intelligence have emerged in the last decades. We now have machine intelligence for drug discoveries and machine intelligence taking on hedge funds. Nearly every contemporary business relies on machine intelligence to solve problems in some way. SpaceX uses machine intelligence to take-off and land their rockets. Facebook uses machine intelligence to determine what you see in your newsfeed. It is almost impossible to think of an industry or market that has not been disrupted by machine intelligence. For all those that have not, it will only be a matter of time.
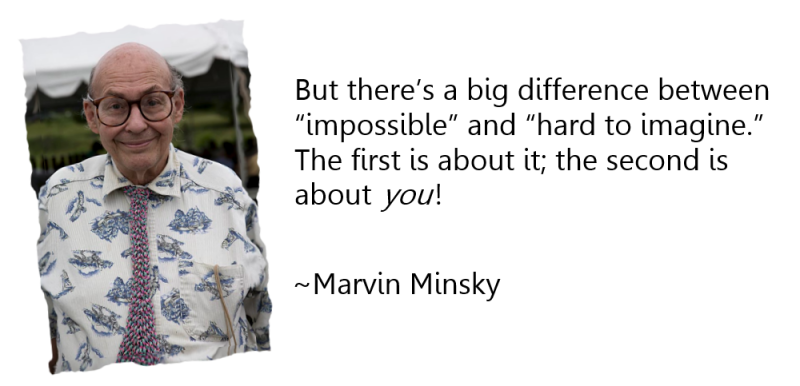
Why Minsky Didn’t Live to See His Dream
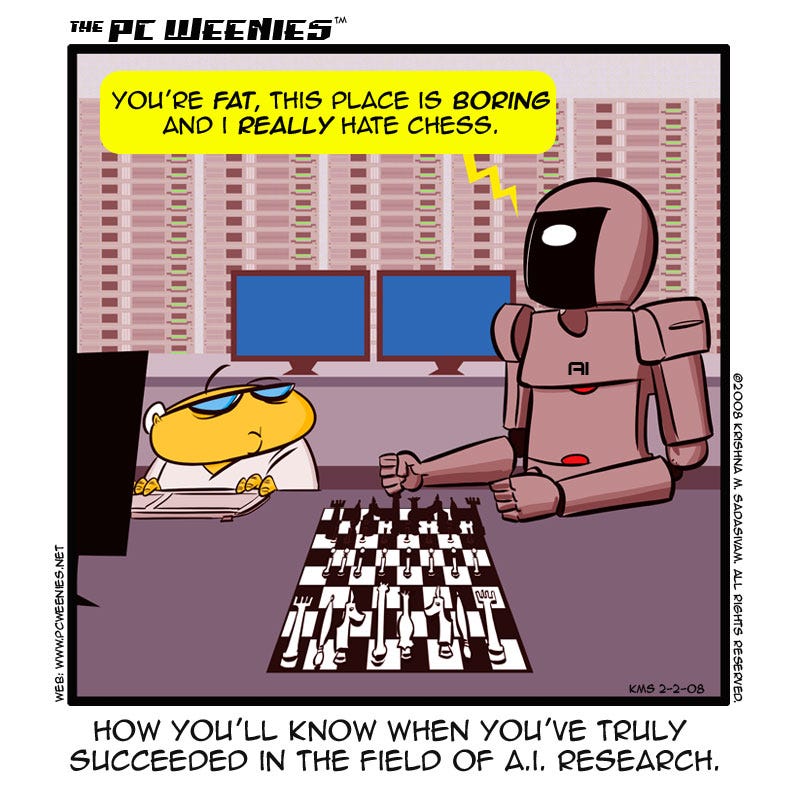
“Today’s artificial-intelligence practitioners seem to be much more interested in building applications that can be sold, instead of machines that can understand stories. The field attracts not as many good people as before.”
Marvin Minsky
Our educational systems have an endemic tendency to teach the most current paradigm as the quintessential foundation on which all progress and innovation has to be built, while neglecting the origins, historical paths and the alternative turns that a research area could have been taken. As a result, not only students, but also most researchers often fail to understand first-principle problems within their field, because those research assumptions have never been presented in a way to be critically reconsidered. For a great book on the history of AI research that provides a detailed history of how we got to the current paradigm, check out Daniel Crevier’s AI: The Tumultuous History of the Search for Artificial Intelligence.
For a broader perspective, Thomas Kuhn attempted to define chronological phases of science in his book The Structure of Scientific Revolutions. He argued that ‘Paradigm Shifts’ or ‘Revolutions’ in scientific research were actually less random than potentially perceived and may depend on a cyclical nature of assumption surfacing and testing that was part of the fundamental nature of science.
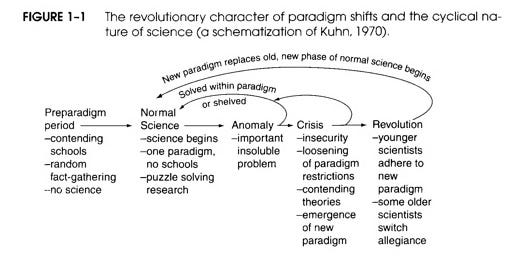
Kuhn’s Phase 1 is an epoch of research that exists only once. This is the so-called ‘pre-paradigm’ phase, in which there is no consensus on any particular theory. This phase is characterized by several incompatible and incomplete theories. For AI, Phase 1 lasted roughly from the 1890s to the 1960s, where the computational perspective on intelligence was explored by thinkers like Charles Babbage, Ada Lovelace, Alan Turing, Dreyfus and Dreyfus, Marvin Minsky and the other participants of the Dartmouth Conference.
Next comes Phase 2—a period of so-called ‘Normal Science’, in which puzzles are solved within the context of the dominant paradigm. Over time, progress in these research areas may reveal anomalies, as well as facts that are difficult to explain within this context. Since the 1970s, AI has largely become a field of theoretical and applied data processing (especially machine learning), knowledge management and robotics. Despite the declining interest in generally capable artificial intelligence, research into ‘narrow AI’ research has been incredibly productive. Expert systems, game playing, theorem provers, self-driving cars and autonomous drones, machine translation, speech recognition and computer vision are some of the many innovations conceived within this realm of ‘normal science’. Yet with respect to understanding broader intelligence, the narrow AI paradigm has been largely unsuccessful.
AI as a science of intelligence is currently in Kuhn’s Phase 3. This is when the phase is a crisis. After significant efforts of normal science within a given paradigm fail, the discipline may be forced to enter the next phase. Minsky considered the efforts of most of his colleagues as insufficient to address intelligence. He pushed against everyone in the field that dared to think too small, while his own school turned away from the trends of neural networks, developmental approaches and perceptual learning. Minsky recognized that AI was pigeon-holing itself in a way that yielded useful applications and short-term results, yet made progress towards the goal of machine intelligence elusive.
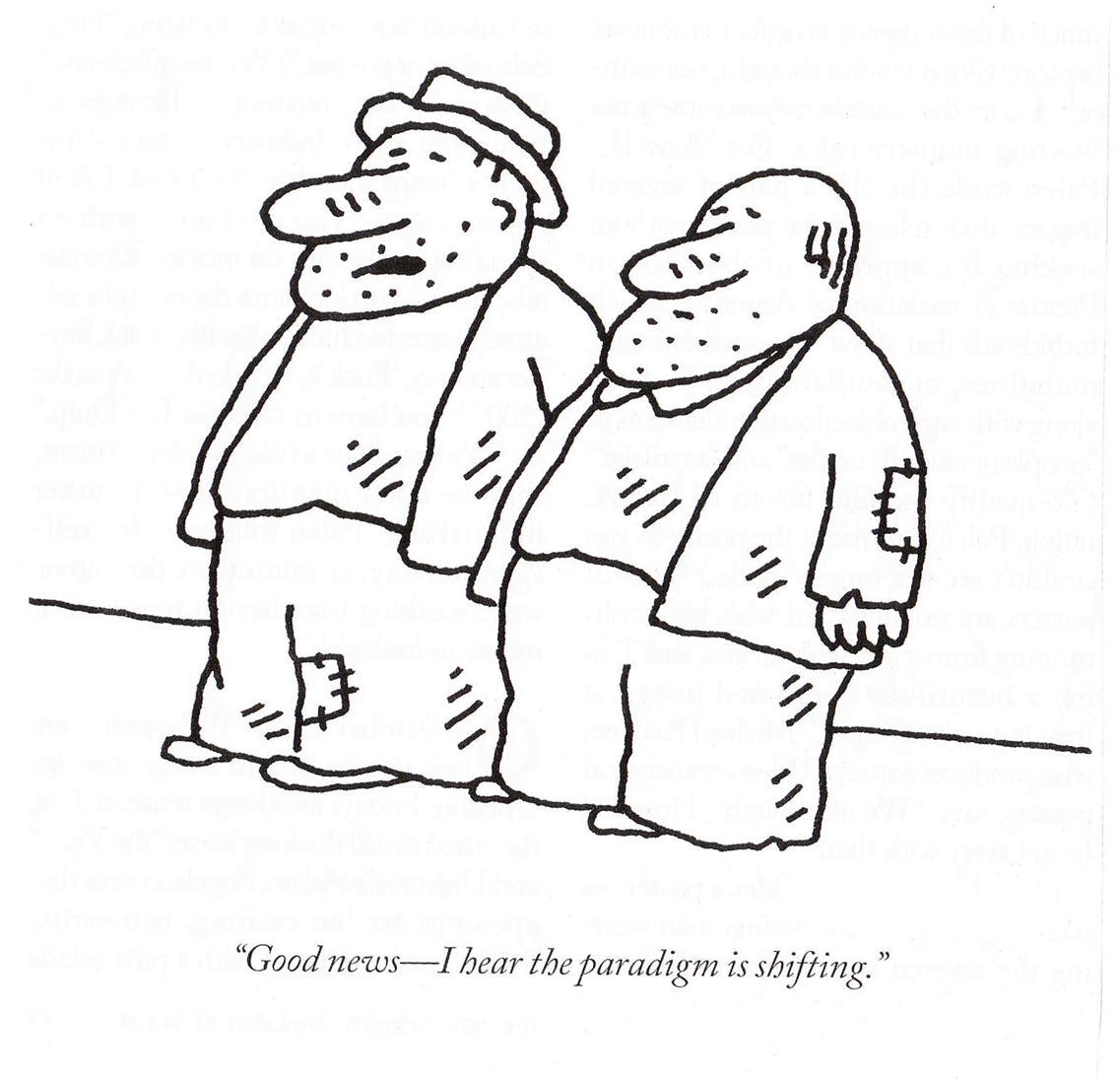
Minsky did not live to see above human-level artificial intelligence because the field he helped to start is in Kuhn’s Phase 3—Crisis Mode. We need to advance AI research to Phase 5: ’Post-Revolution’. Why aren’t more people doing this? Because Phase 4 is really hard. Phase 4 requires us to review and reconsider and partially re-invent an entire research area, which is usually highly unrewarding for an individual with a position in ‘normal science’. It means stepping off the conveyor belt of traditional incentive structures and being prolific. It means going against tradition and most of your peers. It requires the rapid prototyping of ideas. It requires the consideration of a wider spectrum of research including many neighboring fields. It also calls for us to test and critique the assumptions of a widely accepted paradigm, doing away with some of the established methods and processes to allow for a greater proportion of our effort to be spent on the innovation we aim for.
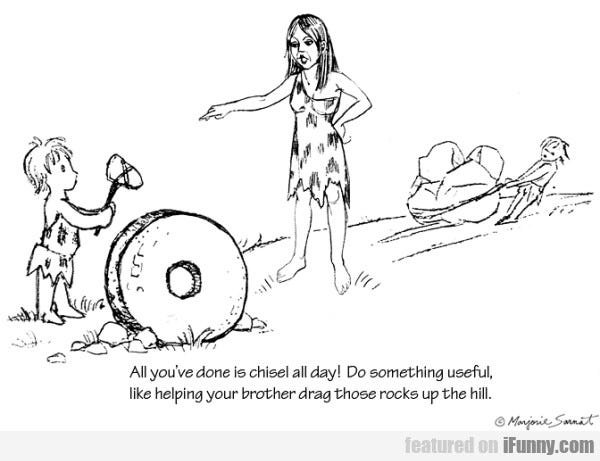
The development of machine intelligence won’t just come from incremental improvements in software that already exists, or by extending current software with greater computational resources. How much of current AI progress is just a demonstration of unique new data sets and unprecedented access to compute? People forget that Reinforcement Learning was an idea put forward by Sutton in the late 1980s, only now looking flashy thanks to data and compute. We need to be thinking bigger. Perhaps we need to reconsider the full stack of computation; perhaps even redefine the entire notion of computing. Where are the much needed spaces to host these discussions?
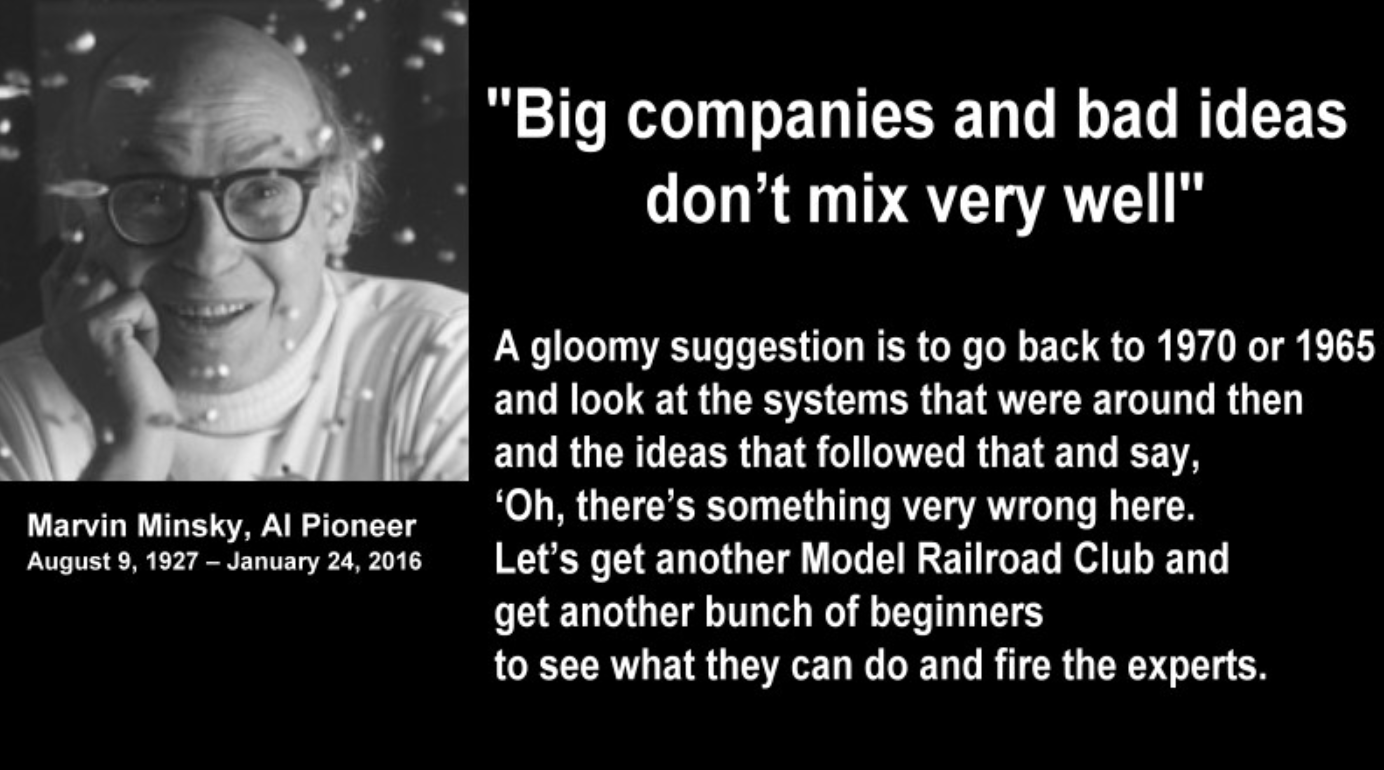
To honor Minsky, we need to be re-thinking artificial intelligence from first principles and not just rely on corporate research teams. We need to be looking at computer science, software development, cognitive science, neuroscience, psychology— and mathematics — with fresh eyes. We need spaces where researchers can develop ideas for long-term goals and where novel ideas can be incubated without the need to fulfill the key performance indicators of the previous paradigm. We need new scientific institutes and to experiment with new research funding vehicles. Progress can’t and won’t only depend on research, it will require others to take the time to build the necessary conditions for these ideas to foster. We need the craziest of ideas to be considered and rewarded, a task that academic research is failing to do. We need academic journals for negative or inconclusive results. We need to reward people trying. We need to be taking much, much more risk.
Minsky understood the scope and the potential reward of trying to pull AI off, yet he died without getting to see it. If only one generation could stop and consider how vast the effects of achieving AI as a meta-science could be. If only we could think from first-principles more regularly, we would all be putting more time, attention and money into busting out AI research from Kuhn’s Phase 3.